Data Strategy Assessment
A Scholarly Approach to Optimizing Data Ecosystems
Introduction
In the contemporary landscape of organizational management, data has emerged as a cornerstone of strategic decision-making, operational efficiency, and innovation. However, the complexity of modern data ecosystems often leads to fragmented strategies, misaligned processes, and unrealized potential, particularly when organizations fail to adopt a systematic, academically grounded approach to data management.
This Data Strategy Assessment (DSA) framework, inspired by Khan, W. (2014)’s seminal Four Stages of Data, offers a rigorous, theoretically informed methodology to evaluate and enhance organizational data strategies.
The framework delineates the data lifecycle into four interconnected layers (i) Acquire and Process, (ii) Visualize, (iii) Interact, and (iv) Retrieve each further dissected, to ensure a comprehensive evaluation. Designed to serve organizations of all scales, from small private firms to large public entities, this assessment integrates principles from data management frameworks such as DAMA-DMBOK, ISO 8000 for data quality, and data maturity models, ensuring a scholarly foundation that balances theoretical depth with practical applicability.
By addressing critical dimensions such as data ethics, cultural alignment, sustainability, and innovation readiness, the assessment empowers organizations to align their data practices with strategic objectives, foster stakeholder trust, and drive long-term success.
Whether you are a small property firm seeking to optimize tenant management, a medium-sized construction company scaling operations, a large corporate managing global projects, or a public entity ensuring civic compliance, this framework offers a pathway to data excellence.
The Four Stages of Data
A Scholarly Foundation for Data Strategy Assessment
The Four Stages of Data framework provides a theoretically robust foundation for understanding the data lifecycle, offering a systematic approach to evaluate and enhance organizational data strategies. Developed through rigorous academic inquiry, this framework delineates the data lifecycle into four interconnected layers, each serving as a critical pillar for assessing data strategy readiness.
By providing a holistic lens, the framework empowers organizations to develop a strategic roadmap that not only addresses current deficiencies but also positions them for long-term success in an increasingly data-driven global landscape.
1. Acquire and Process
The Acquire and Process stage constitutes the foundational layer of any data strategy, focusing on the collection, cleaning, and enrichment of raw data to ensure its quality and usability for downstream applications.
From a data strategy assessment perspective, this stage evaluates the mechanisms through which organizations gather data, such as IoT sensors on construction sites or tenant records in property management, ensuring that these processes align with international standards for data quality, such as those outlined by ISO 8000.
It also examines the extent to which data is enriched with contextual metadata, enabling advanced analytics and ensuring relevance to strategic objectives, such as optimizing project costs or enhancing tenant satisfaction.
From an academic standpoint, this stage further assesses the ethical and cultural dimensions of data acquisition, such as the fairness of sourcing practices and the inclusivity of data representation, which are critical for organizations operating in diverse contexts.
A public sector entity managing civic infrastructure, for instance, must ensure that its data collection practices adhere to ethical standards like GDPR, while a medium-sized construction firm might evaluate whether its data sources reflect regional cultural nuances, such as local building codes.
By incorporating dimensions like sustainability tracking (e.g., capturing carbon emissions data) and innovation readiness (e.g., adopting AI for data validation), this stage ensures that organizations build a data foundation that is technically sound, ethically responsible, and strategically aligned with long-term goals.
2. Visualize
The Visualize stage transforms processed data into actionable insights through visual representations, simulations, and predictive models, serving as a pivotal juncture in the data strategy where raw information is rendered meaningful for decision-making.
From a data strategy assessment perspective, this stage evaluates the clarity, timeliness, and strategic relevance of visualizations, ensuring they empower stakeholders to make informed decisions in dynamic environments, such as a small property firm visualizing occupancy trends or a large corporate simulating cost overruns for global projects.
Beyond technical efficacy, the Visualize stage also examines the socio-ethical implications of data presentation, such as the avoidance of bias in visual representations and the integration of sustainability metrics, which are increasingly vital in property and construction contexts.
By incorporating dimensions like innovation readiness (e.g., the use of AR/VR for immersive visualizations) and stakeholder inclusivity (e.g., ensuring accessibility for non-technical users), this stage ensures that visualizations are not only informative but also ethically sound and culturally aligned.
3. Interact
The Interact stage focuses on user engagement with data systems, prioritizing accessibility, collaboration, and system interoperability to foster effective teamwork and ensure data is actionable across diverse organizational levels.
From a data strategy assessment perspective, this stage examines the ease of access to data systems, the effectiveness of collaborative workflows, and the integration of disparate systems, ensuring seamless usability in operational contexts.
A global retail business might assess whether its sales teams can access customer data seamlessly across regions, ensuring alignment with marketing strategies. Similarly, a local authority could evaluate the inclusivity of its public service portal, ensuring accessibility for residents with disabilities.
By integrating dimensions such as innovation readiness (e.g., leveraging AI-driven collaboration tools) and sustainability integration (e.g., linking systems to monitor energy usage in public facilities), this stage ensures that data system interactions are efficient, ethically sound, and strategically aligned with organizational goals.
4. Retrieve
Fundamentally, the Retrieve stage ensures that data remains accessible, secure, and compliant over extended periods, enabling long-term planning, regulatory adherence, and historical analysis. From a data strategy assessment perspective, this stage examines the precision and speed of retrieval processes, assessing how efficiently organizations can access critical data, such as operational logs or compliance reports, when required.
It also evaluates the reliability of archival systems, ensuring that data integrity is preserved over time, which is vital for organizations to meet audit requirements or inform strategic decisions.
Equally, the Retrieve stage delves into the socio-ethical dimensions of data management, emphasizing the ethical transparency of governance practices and their alignment with societal expectations.
A global logistics firm, for instance, might assess whether its governance policies provide clear visibility into data usage for international trade regulators, fostering trust and accountability.
Moreover, the sustainability dimensions of data retrieval are prioritized, especially as environmental impact becomes a pressing concern. This stage evaluates the environmental footprint of archival systems, such as the energy consumption of data centers used for long-term storage, encouraging organizations to adopt more sustainable practices.
A regional arts council, for example, might assess whether its archival infrastructure uses energy-efficient technologies to store digital records of cultural performances, aligning with environmental goals.
Focusing on sustainability ensures that data retrieval practices contribute to broader ecological objectives, reflecting a commitment to responsible resource management.
Lastly, the Retrieve stage integrates dimensions such as innovation readiness and cultural alignment to ensure that data retrieval practices are forward-thinking and contextually relevant.
A multinational e-commerce company might explore the use of AI for predictive retrieval, anticipating future data needs for customer behavior analysis, while a local historical society could evaluate whether its archival practices preserve culturally significant records, such as oral histories from marginalized communities.
Incorporating these dimensions ensures that data retrieval is technically robust, ethically responsible, and strategically aligned with organizational priorities and societal needs, fostering a data strategy that is both resilient and inclusive.
To enhance the framework’s academic rigor and practical utility, each layer has been expanded with additional sub-layers and sub-sub-layers, incorporating dimensions such as data ethics (e.g., ethical sourcing), cultural alignment (e.g., stakeholder inclusivity), sustainability integration (e.g., environmental data tracking), and innovation readiness (e.g., AI adoption). This multi-tiered approach ensures a holistic assessment of data strategy readiness, addressing both technical and socio-ethical dimensions of data management, and providing a comprehensive foundation for strategic roadmapping.
The Four Stages of Data framework, as originally proposed by Khan (2014), offers a theoretically grounded lens for assessing data strategy readiness, enabling organizations to navigate the complexities of modern data ecosystems with precision and foresight.
The Need for Data Strategy Assessment
The exponential growth of organizational data presents significant opportunities alongside operational and strategic challenges. Entities across sectors encounter distinct obstacles: small firms face resource constraints, medium-sized organizations grapple with scalability, large corporations contend with fragmented data ecosystems, and public institutions must navigate stringent regulatory demands. A Data Strategy Assessment offers a structured, theoretically grounded methodology to address these issues by:
A. Identifying Systemic Deficiencies
Applying systems thinking to detect inefficiencies across data workflows—from collection to utilization—that impede organizational objectives.
B. Ensuring Strategic Coherence
Aligning data governance with business goals, such as operational optimization or stakeholder satisfaction, informed by strategic alignment theory.
C. Upholding Ethical and Regulatory Compliance
Embedding principles from data ethics frameworks to meet legal standards (e.g., GDPR, industry-specific regulations).
D. Advancing Innovation and Sustainable Practices
Facilitating the integration of emerging technologies (e.g., AI, IoT) and sustainability metrics (e.g., emissions tracking) through interdisciplinary insights from sustainability science.
E. Strengthening Stakeholder and Cultural Relevance
Incorporating cultural informatics and stakeholder theory to ensure data systems are inclusive and contextually appropriate.
F. Delivering Adaptable Frameworks
Providing scalable solutions tailored to diverse organizational sizes and sectors, from startups to multinational enterprises.
Rooted in academic rigor, this assessment equips organizations with a forward-looking roadmap, enabling them to harness data as a strategic asset while mitigating risks in an increasingly data-centric environment.
A Comprehensive Framework for Data Strategy Assessment
In the pursuit of robust data-driven decision-making, organizations must strategically (i) acquire and process data to ensure its reliability and relevance, (ii) visualize it to uncover actionable insights, (iii) interact with it to foster collaboration and innovation, and (iv) retrieve it efficiently to support operational and strategic objectives.
This academic framework provides a structured approach to evaluating data strategy readiness, emphasizing qualitative dimensions such as technical robustness, operational efficiency, strategic alignment, ethical compliance, cultural relevance, and innovation potential.
(I). Acquire and Process
The Acquire and Process phase establishes the bedrock of an effective data strategy by ensuring data is systematically collected, refined, and prepared for downstream applications. This phase encompasses three critical dimensions: Data Alignment, Data Integrity, and Context Enhancement.
(I.1) Data Alignment
Data Alignment ensures that data sources are strategically selected and managed to support organizational objectives. This dimension emphasizes the coherence between data acquisition strategies and overarching goals, such as operational efficiency or stakeholder satisfaction. Key assessment areas include:
(I.1.1.) - Source Relevance
Evaluating the degree to which data sources—such as real-time sensor feeds or market intelligence—directly contribute to strategic priorities, such as cost reduction or enhanced customer engagement.
(I.1.2.) - Scalability Capacity
Assessing the infrastructure’s ability to accommodate growing data demands, ensuring systems remain robust as organizational needs evolve.
(I.1.3.) - Ethical Sourcing
Verifying adherence to ethical standards, including compliance with data protection regulations (e.g., GDPR) and equitable sourcing practices that respect stakeholder rights.
(I.1.4.) - Cultural Alignment
Examining whether data sources account for the cultural and contextual diversity of stakeholders, such as incorporating multilingual datasets to support global operations.
(I.1.5.) - Innovation Readiness
Investigating the integration of cutting-edge technologies, such as Internet of Things (IoT) devices or blockchain, to enhance the precision and scope of data collection.
(I. 2) Data Integrity
Data Integrity focuses on maintaining the accuracy, reliability, and trustworthiness of data throughout its lifecycle. This dimension ensures that data remains a credible foundation for decision-making. Key assessment areas include:
(I.2.1) - Accuracy Assurance
Analyzing mechanisms for identifying and rectifying errors, such as inconsistencies in operational records or transactional datasets, to uphold data quality.
(I.2.2) - Validation Efficiency
Evaluating the speed and automation of validation processes, ensuring timely availability of reliable data without compromising rigor.
(I.2.3) - Auditability
Assessing the presence of comprehensive audit trails to promote transparency and enable traceability, critical for regulatory compliance and accountability.
(I.2.4) - Sustainability Tracking
Investigating the incorporation of environmental metrics, such as carbon footprint data, into validation processes to align with sustainability goals.
(I.2.5) - Data Ethics
Ensuring that data validation practices mitigate risks of bias or manipulation, fostering fairness and integrity in data handling.
(I. 3) Context Enhancement
Context Enhancement enriches raw data with metadata to maximize its usability and analytical potential. This dimension ensures that data is not only accurate but also meaningfully structured for diverse applications. Key assessment areas include:
(I.3.1) - Metadata Richness
Evaluating the comprehensiveness and utility of metadata, such as annotations linking datasets to specific project milestones or operational contexts.
(I.3.2) - Contextual Automation
Assessing the deployment of advanced tools, such as artificial intelligence or natural language processing, to streamline metadata generation and improve efficiency.
(I.3.3) - Semantic Utility
Investigating the extent to which metadata enables sophisticated analytical capabilities, such as supporting complex semantic queries for predictive modeling.
(I.3.4) - Stakeholder Inclusivity
Ensuring metadata is designed to be accessible and relevant to diverse user groups, including compliance with accessibility standards for equitable use.
(I.3.5) - Cultural Relevance
Verifying that metadata reflects local or regional nuances, such as jurisdictional requirements or cultural preferences, to enhance its applicability.
The Acquire and Process phase guarantees data is thoroughly gathered, refined, and aligned with organizational goals—ensuring compliance and effectiveness across technical, ethical, and cultural dimensions.
(II). Visualize
The Visualize phase transforms raw data into actionable insights, enabling evidence-based decision-making across organizational contexts. This phase encompasses three critical dimensions: Decision Mapping, Metric Precision, and Impact Simulation.
(II.1) Decision Mapping
Decision Mapping leverages visual representations to facilitate strategic and operational decision-making. This dimension emphasizes the clarity, timeliness, and impact of visualizations in supporting diverse stakeholders. Key assessment areas include:
(II.1.1.) - Visual Clarity
Evaluating the extent to which visualizations, such as dashboards or reports, are intuitive and comprehensible for stakeholders like project managers or investors.
(II.1.2.) - Update Frequency
Assessing the timeliness of data refreshes in visualizations to meet decision-making needs, such as real-time versus daily updates.
(II.1.3.) - Decision Impact
Investigating how visualizations contribute to improved outcomes, such as reducing project delays or optimizing resource allocation.
(II.1.4.) - Innovation Readiness
Exploring the adoption of advanced visualization technologies, such as augmented reality for virtual site walkthroughs.
(II.1.5.) - Sustainability Visualization
Verifying the integration of environmental metrics, such as energy consumption, into visualizations to support sustainability goals.
(II.2) Metric Precision
Metric Precision ensures that visualized data accurately reflects underlying realities, fostering trust and reliability. This dimension focuses on the fidelity and performance of visualization systems. Key assessment areas include:
(II.2.1.) - Data Accuracy
Analyzing the alignment between visualized metrics and verified data sources, such as actual versus reported project costs.
(II.2.2.) - Latency Impact
Evaluating the responsiveness of visualization systems under varying data loads to ensure consistent performance.
(II.2.3.) - Stakeholder Trust
Assessing the confidence that stakeholders place in the reliability of visualized metrics.
(II.2.4.) - Cultural Alignment
Ensuring visualizations are tailored to cultural preferences, such as using locally relevant units of measurement.
(II.2.5.) - Data Ethics
Examining visualization practices to prevent the reinforcement of biases, such as equitable representation in analytics.
(II.3) Impact Simulation
Impact Simulation enables organizations to model potential outcomes, supporting proactive strategic planning. This dimension emphasizes the robustness and inclusivity of simulation tools. Key assessment areas include:
(II.3.1.) - Model Robustness
Evaluating the accuracy and reliability of simulation models across diverse scenarios.
(II.3.2.) - Scenario Coverage
Assessing the comprehensiveness of scenarios modeled, such as market fluctuations or cost overruns.
(II.3.3.) - Strategic Alignment
Investigating the extent to which simulations support organizational priorities, such as sustainability goals.
(II.3.4.) - Innovation Readiness
Exploring the integration of artificial intelligence-driven tools to enhance simulation accuracy.
(II.3.5.) - Stakeholder Inclusivity
Ensuring simulation outputs are accessible to non-technical stakeholders through simplified interfaces.
(III). Interact
The Interact phase ensures that users can engage with data systems effectively, fostering collaboration, accessibility, and seamless integration. This phase encompasses three critical dimensions: User Access, Collaboration, and Integration.
(III.1) User Access
User Access facilitates efficient and inclusive engagement with data systems, ensuring usability across diverse contexts. This dimension emphasizes the ease, compatibility, and fairness of access mechanisms. Key assessment areas include:
(III.1.1.) - Accessibility Ease
Evaluating the efficiency of access mechanisms, such as login processes or navigation interfaces, for streamlined user experiences.
(III.1.2.) - Device Compatibility
Assessing the system’s support for a range of devices, including mobile phones, tablets, and desktops, to ensure broad accessibility.
(III.1.3.) - Inclusive Design
Investigating compliance with accessibility standards, such as WCAG, to accommodate users with disabilities.
(III.1.4.) - Cultural Alignment
Examining whether access mechanisms incorporate culturally appropriate features, such as multilingual interfaces or region-specific formats.
(III.1.5.) - Innovation Readiness
Exploring the adoption of advanced access technologies, such as biometric authentication, to enhance security and usability.
(III.2) Collaboration
Collaboration enables effective team interactions through shared data systems, promoting efficiency and inclusivity. This dimension focuses on the performance and adoption of collaborative tools. Key assessment areas include:
(III.2.1.) - Sync Efficiency
Assessing the reliability of collaborative workflows, such as real-time updates to shared documents or datasets.
(III.2.2.) - Real-Time Capability
Evaluating the performance of tools enabling real-time collaboration, ensuring minimal latency and high responsiveness.
(III.2.3.) - Team Adoption
Investigating the extent to which teams actively engage with and utilize collaboration tools for project coordination.
(III.2.4.) - Innovation Readiness
Exploring the integration of advanced collaboration technologies, such as AI-driven facilitators or digital whiteboards, to enhance teamwork.
(III.2.5.) - Data Ethics
Ensuring that collaboration tools promote equitable access and prevent exclusion across diverse user groups.
(III.3) Integration
Integration ensures seamless interoperability among data systems, supporting scalability and coherence. This dimension emphasizes the connectivity and reliability of integrated platforms. Key assessment areas include:
(III.3.1.) - System Interoperability
Evaluating the connectivity between systems, such as CRM and ERP platforms, to enable cohesive data flows.
(III.3.2.) - Error Frequency
Assessing the reliability of data exchanges between systems, minimizing disruptions or inconsistencies.
(III.3.3.) - Scalability Support
Investigating the capacity of integrated systems to accommodate new platforms as organizational needs evolve.
(III.3.4.) - Sustainability Integration
Examining the incorporation of environmental metrics, such as energy usage data, into integrated systems to support sustainability goals.
(III.3.5.) - Cultural Alignment
Ensuring that integrated systems accommodate diverse user requirements, such as regional data formats or standards.
(IV). Retrieve
The Retrieve phase ensures that data remains accessible, secure, and compliant over time, supporting organizational needs and stakeholder trust. This phase encompasses four critical dimensions: Accuracy, Speed, Record, and Governance.
(IV.1) Accuracy
Accuracy ensures the precision and reliability of retrieved data, fostering confidence in its use. This dimension emphasizes the correctness and fairness of retrieval processes. Key assessment areas include:
(IV.1.1.) - Retrieval Precision
Evaluating the accuracy of retrieved data, such as ensuring correct permit records are accessed.
(IV.1.2.) - Error Detection
Assessing mechanisms for identifying and correcting errors during data retrieval.
(IV.1.3.) - User Confidence
Investigating the level of stakeholder trust in the reliability of retrieved data.
(IV.1.4.) - Innovation Readiness
Exploring the adoption of artificial intelligence to enhance the accuracy of data retrieval.
(IV.1.5.) - Data Ethics
Examining whether retrieval processes mitigate biases to ensure equitable access to data.
(IV.2) Speed
Speed optimizes the performance of data retrieval, ensuring timely access under varying conditions. This dimension focuses on the efficiency and sustainability of retrieval systems. Key assessment areas include:
(IV.2.1.) - Retrieval Latency
Assessing the efficiency and responsiveness of data retrieval processes.
(IV.2.2.) - Indexing Efficiency
Evaluating the performance of indexing mechanisms to facilitate rapid data access.
(IV.2.3.) - Load Handling
Investigating the system’s ability to maintain performance during peak demand periods.
(IV.2.4.) - Innovation Readiness
Exploring the use of predictive caching technologies to enhance retrieval speed.
(IV.2.5.) - Sustainability Archiving
Assessing the energy efficiency of retrieval systems to align with environmental goals.
(IV.3) Record
Record ensures the completeness and reliability of data archives, preserving information for long-term use. This dimension emphasizes the integrity and cultural relevance of archival practices. Key assessment areas include:
(IV.3.1.) - Data Completeness
Evaluating the extent to which records are fully preserved over time.
(IV.3.2.) - Retrieval Reliability
Assessing the success rate of accessing archived data without loss or corruption.
(IV.3.3.) - Retention Compliance
Investigating adherence to data retention policies and regulatory requirements.
(IV.3.4.) - Sustainability Archiving
Examining the inclusion of environmental data, such as energy usage records, in archives.
(IV.3.5.) - Cultural Alignment
Assessing whether archival practices preserve culturally significant data, such as historical property records.
(IV.4) Governance
Governance ensures compliance with data policies, promoting transparency and inclusivity. This dimension focuses on the robustness and ethical alignment of governance frameworks. Key assessment areas include:
(IV.4.1.) - Policy Coverage
Evaluating the comprehensiveness of data governance policies across organizational operations.
(IV.4.2.) - Enforcement Effectiveness
Assessing the strength of mechanisms for enforcing data governance policies.
(IV.4.3.) - Ethical Transparency
Investigating the transparency of data practices to build stakeholder trust.
(IV.4.4.) - Stakeholder Inclusivity
Examining whether governance processes incorporate diverse stakeholder perspectives, such as community input.
(IV.4.5.) - Data Ethics
Assessing alignment with ethical principles, such as fairness and accountability, in data management.
Methodology
The assessment process is grounded in academic rigor, drawing on data management frameworks (e.g., DAMA-DMBOK, ISO 8000), systems thinking, and ethical data governance principles. The methodology includes:
1. Data Collection Gather qualitative and quantitative data through stakeholder interviews, system audits, and process reviews to assess each sub-sub-layer.
2. Readiness Evaluation Conduct a qualitative analysis of readiness across technical, operational, strategic, ethical, cultural, and innovation dimensions, focusing on strengths, weaknesses, and opportunities.
3. Gap Analysis Identify systemic gaps in data strategy, such as misaligned data sources, inefficient retrieval processes, or lack of ethical transparency.
4. Strategic Roadmapping Develop a tailored roadmap with actionable recommendations, including short-term actions (e.g., automate metadata generation), medium-term goals (e.g., adopt AI-driven simulations), and long-term strategies (e.g., establish a data ethics board).
5. Iterative Review Implement a cyclical review process to monitor progress, adapt to emerging challenges, and ensure continuous improvement.
This methodology ensures a holistic, theoretically informed assessment that balances technical precision with socio-ethical considerations, making it suitable for diverse organizational contexts.
Data Value Example
The expanded Data Strategy Assessment offers transformative value, tailored to the unique needs of organizations across the spectrum:
Small Firms (e.g., Local Property Management)
- Streamline data with automated validation processes, reducing manual errors.
- Enhance decision-making culturally relevant dashboards that reflect local market needs.
- Ensure compliance with local regulations through ethical data sourcing practices.
- Foster inclusivity by adopting accessible data systems for diverse tenants.
Medium-Sized Enterprises (e.g., Regional Construction Company)
- Scale operations by integrating scalable data systems that support growth.
- Adopt AI-driven simulations to plan projects more effectively, reducing risks.
- Track sustainability metrics, aligning with green building standards.
- Promote cultural alignment by ensuring data systems support regional teams.
Large Corporates (e.g., Global Construction Conglomerate)
- Unify global data systems to ensure consistency across regions.
- Leverage AR/VR for advanced project visualization, enhancing stakeholder engagement.
- Mitigate risks through robust governance and ethical transparency.
- Drive innovation by adopting AI and IoT for data collection and analysis.
Public Sector Entities (e.g., Municipal Property Authority)
- Ensure compliance with stringent regulations through comprehensive governance.
- Enhance public service delivery with real-time urban planning simulations.
- Foster trust with transparent, ethical data practices that involve community input.
- Integrate sustainability metrics to support civic environmental goals.
Private Firms (e.g., Property Development Startup)
- Innovate with predictive analytics to forecast market trends, gaining a competitive edge.
- Improve team collaboration with advanced tools like digital whiteboards.
- Support inclusivity with accessible data systems for diverse stakeholders.
- Align data practices with cultural priorities, such as local property regulations.
Scenarios in Real-World Contexts
The Scenarios in Real-World Contexts section illustrates the application of the Data Strategy Assessment framework across diverse organizational settings. This section encompasses four scenarios: Small Property Firm, Medium-Sized Construction Company, Large Corporate, and Public Entity.
Small Property Firm
Small Property Firm represents a local rental business managing 50 properties, aiming to enhance tenant satisfaction and regulatory compliance. The assessment identifies critical gaps and proposes actionable solutions. Key findings include:
Acquire and Process Challenges
Manual data entry for tenant records results in inconsistencies, undermining data reliability (Data Integrity: Accuracy Assurance), and the absence of ethical sourcing practices risks non-compliance (Data Alignment: Ethical Sourcing).
Action Plan
Implement artificial intelligence-driven validation to minimize errors and adopt a blockchain-based platform to ensure ethical data sourcing.
Outcome
The firm achieves reliable tenant records, complies with local data privacy regulations, and strengthens tenant trust.
Medium-Sized Construction Company
Medium-Sized Construction Company focuses on a regional firm building commercial complexes, seeking to scale operations while prioritizing sustainability. The assessment highlights visualization deficiencies and recommends targeted improvements. Key findings include:
Visualize Challenges
Cost simulations lack comprehensive scenario coverage, limiting risk mitigation (Impact Simulation: Scenario Coverage), and environmental metrics are not integrated into dashboards (Decision Mapping: Sustainability Visualization).
Action Plan
Deploy artificial intelligence-driven simulations to address diverse scenarios, such as material price fluctuations, and incorporate energy consumption data into visualization tools.
Outcome
The firm reduces cost overruns by 15%, aligns with green building standards, and secures a sustainability certification.
Large Corporate
Large Corporate describes a multinational construction firm managing global projects, aiming to unify data systems and reduce risks. The assessment reveals interaction barriers and proposes integrative solutions. Key findings include:
Interact Challenges
Siloed systems impede cross-regional collaboration (Integration: System Interoperability), and collaboration tools lack real-time functionality (Collaboration: Real-Time Capability).
Action Plan
Introduce middleware to enhance system interoperability and implement real-time collaboration tools, such as digital whiteboards.
Outcome
The firm improves global coordination, reduces project delays by 20%, and enhances operational efficiency, yielding significant cost savings.
Public Entity
Public Entity represents a municipal authority overseeing public housing, striving to improve service delivery and transparency. The assessment uncovers retrieval and governance gaps, recommending strategic enhancements. Key findings include:
Retrieve Challenges
Governance practices lack transparency, eroding public trust (Governance: Ethical Transparency), and slow retrieval processes delay civic planning (Speed: Retrieval Latency).
Action Plan
Publish public-facing data ethics reports and upgrade retrieval infrastructure with predictive caching technologies.
Outcome
The authority enhances public trust, secures community support for housing initiatives, and accelerates planning processes, improving service delivery.
Get Started with Your Data Strategy Assessment
The Data Strategy Assessment offers an academically grounded framework to align data practices with organizational objectives, ensuring technical robustness, ethical compliance, and strategic coherence. Key steps include:
Consultation
Schedule a consultation to explore your organization’s unique needs and challenges.
Assessment
Conduct a comprehensive evaluation of data practices across all dimensions and components.
Reporting
Receive a detailed report with gap analysis and a tailored strategic roadmap.
Implementation
Execute recommendations and monitor progress through iterative reviews.
Contact Us: Email hello@caspia.co.uk or call +44 784 676 8083 to begin optimizing your data ecosystem.
We're Here to Help!
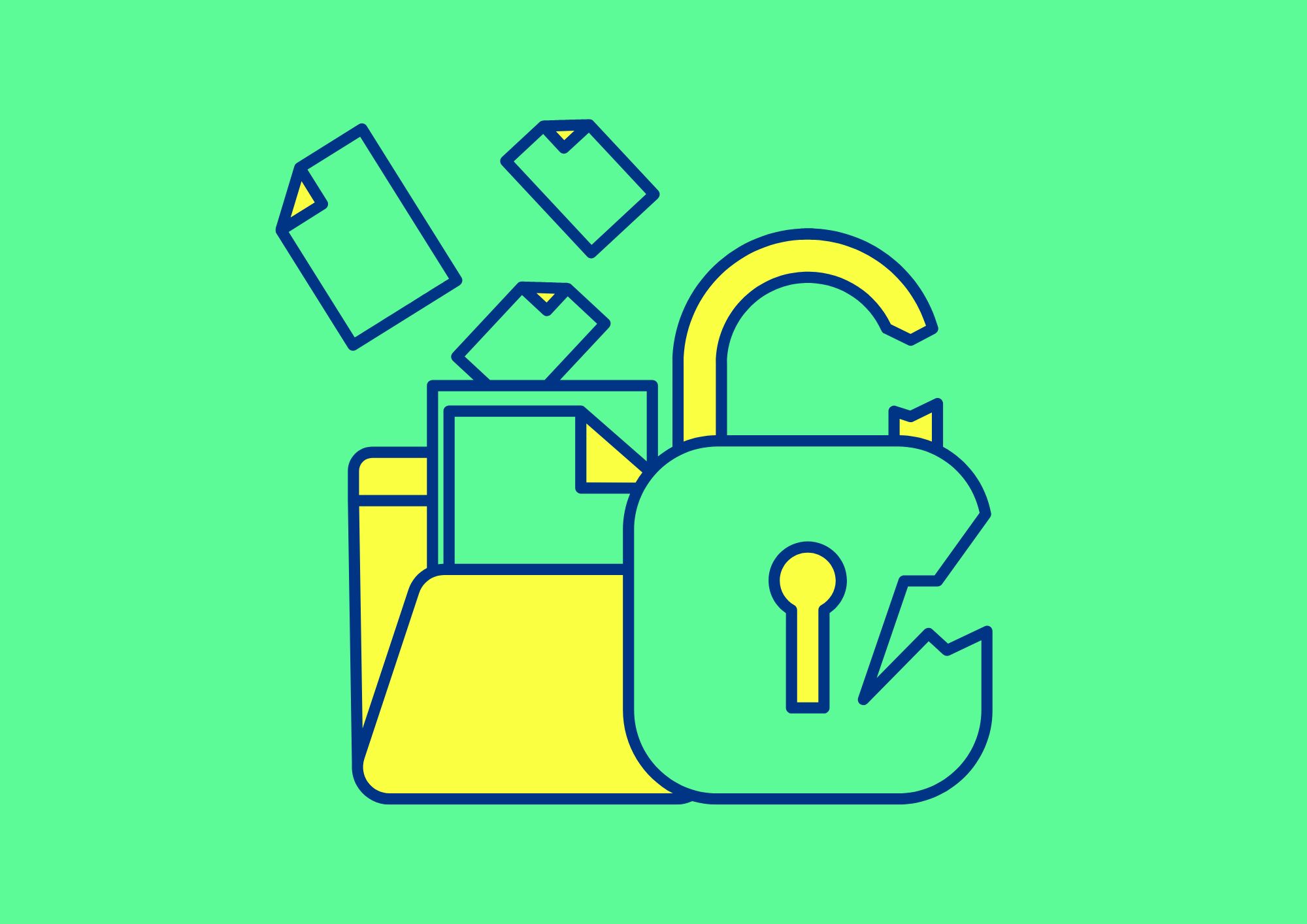
Data Security
Safeguard your data with our four-stage supervision and assessment framework, ensuring robust, compliant, and ethical security practices for resilient organizational trust and protection.
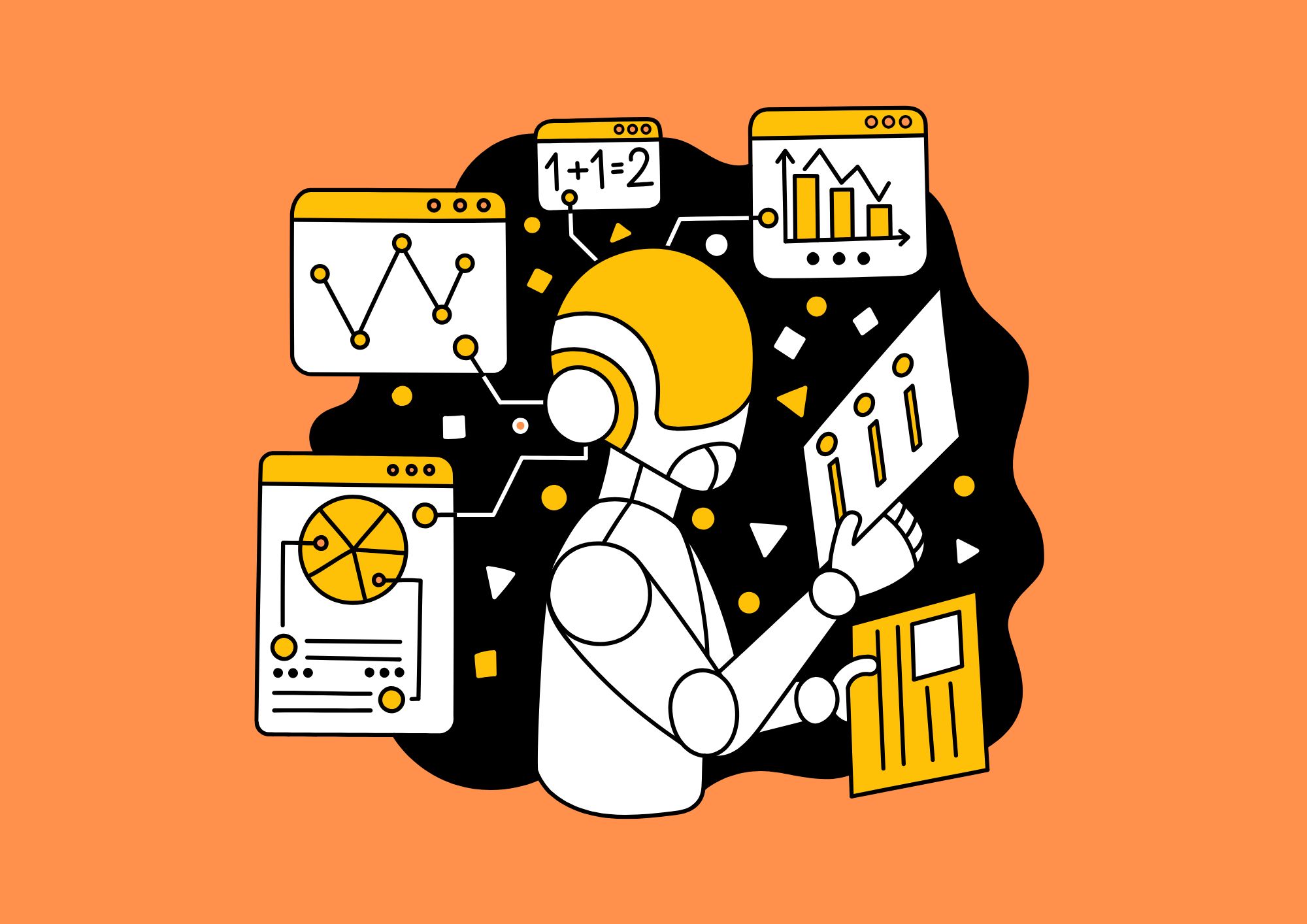
Data and Machine Learning
Harness the power of data and machine learning with our four-stage supervision and assessment framework, delivering precise, ethical, and scalable AI solutions for transformative organizational impact.
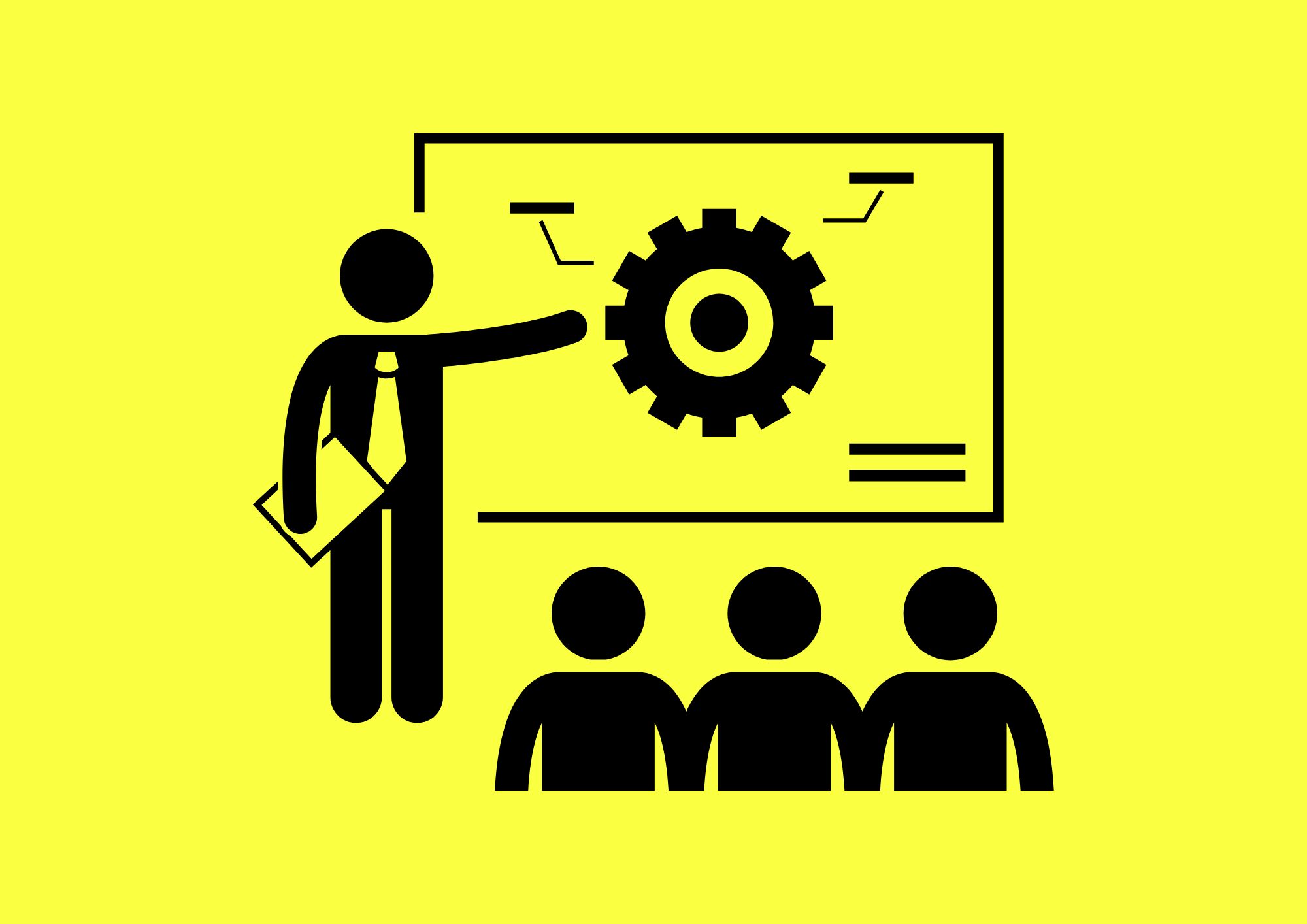
AI Data Workshops
Empower your team with hands-on AI data skills through our four-stage workshop framework, ensuring practical, scalable, and ethical AI solutions for organizational success.
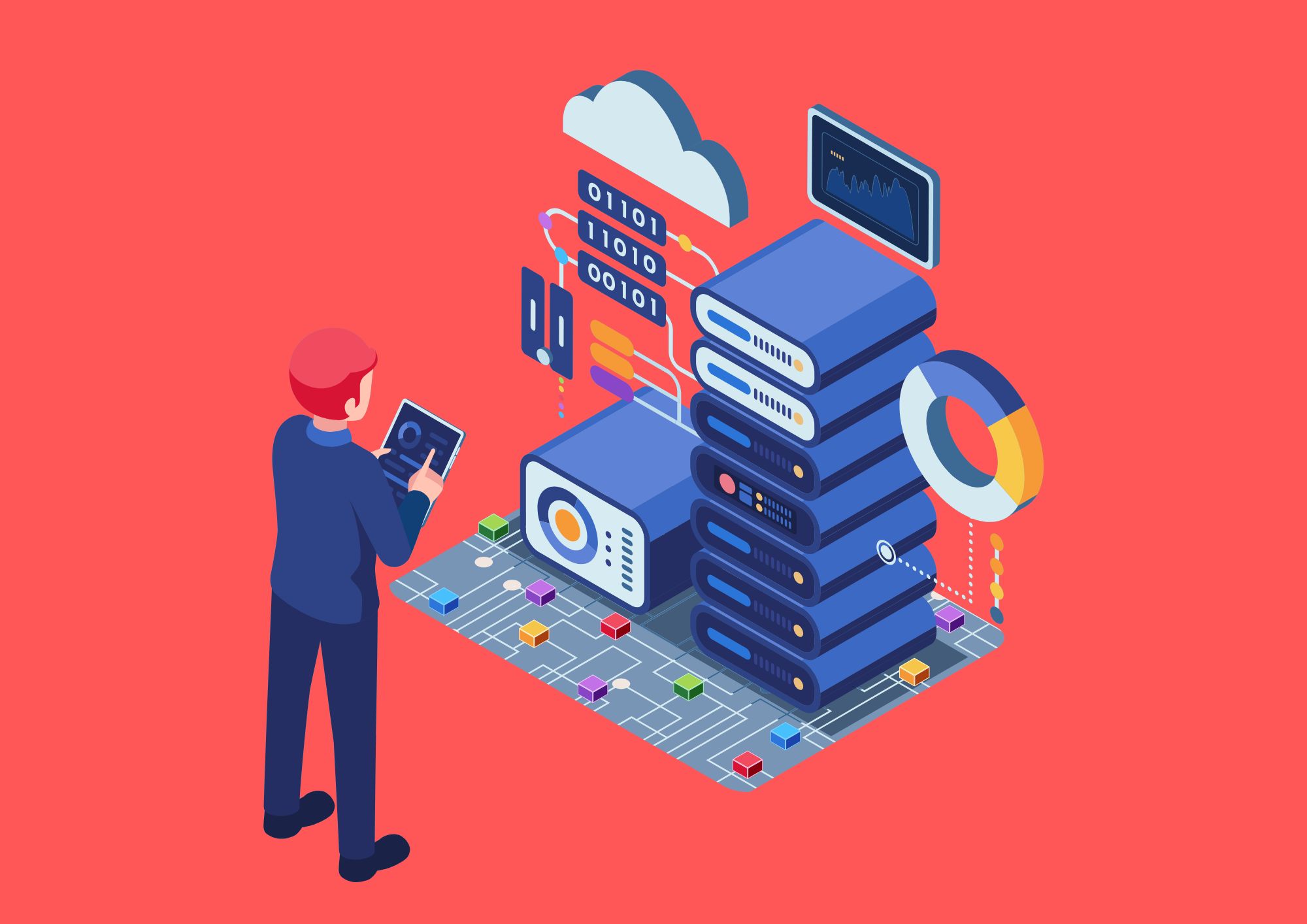
Data Engineering
Architect and optimize robust data platforms with our four-stage supervision and assessment framework, ensuring scalable, secure, and efficient data ecosystems for organizational success.
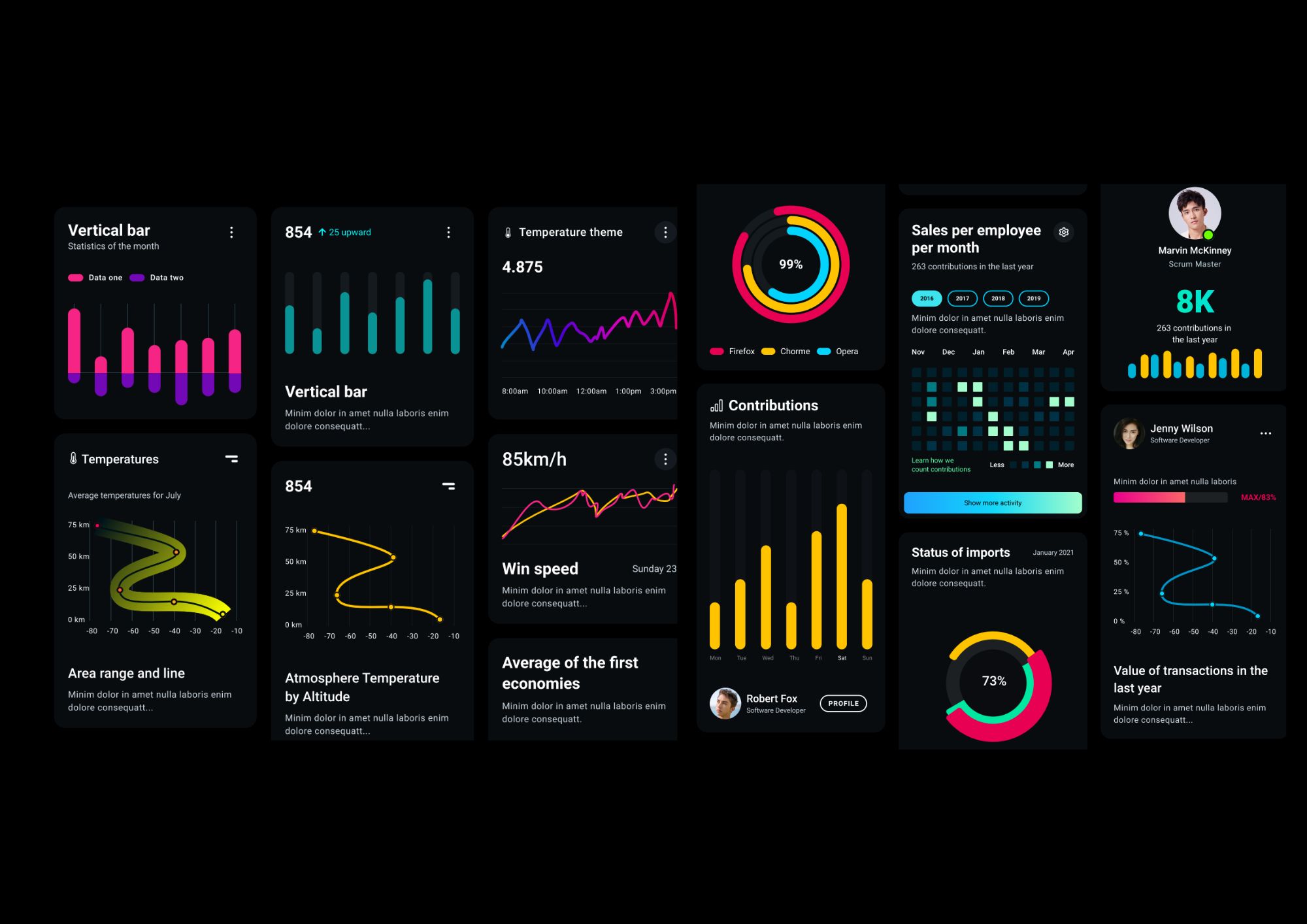
Data Visualization
Harness the power of visualization charts to transform complex datasets into actionable insights, enabling evidence-based decision-making across diverse organizational contexts.
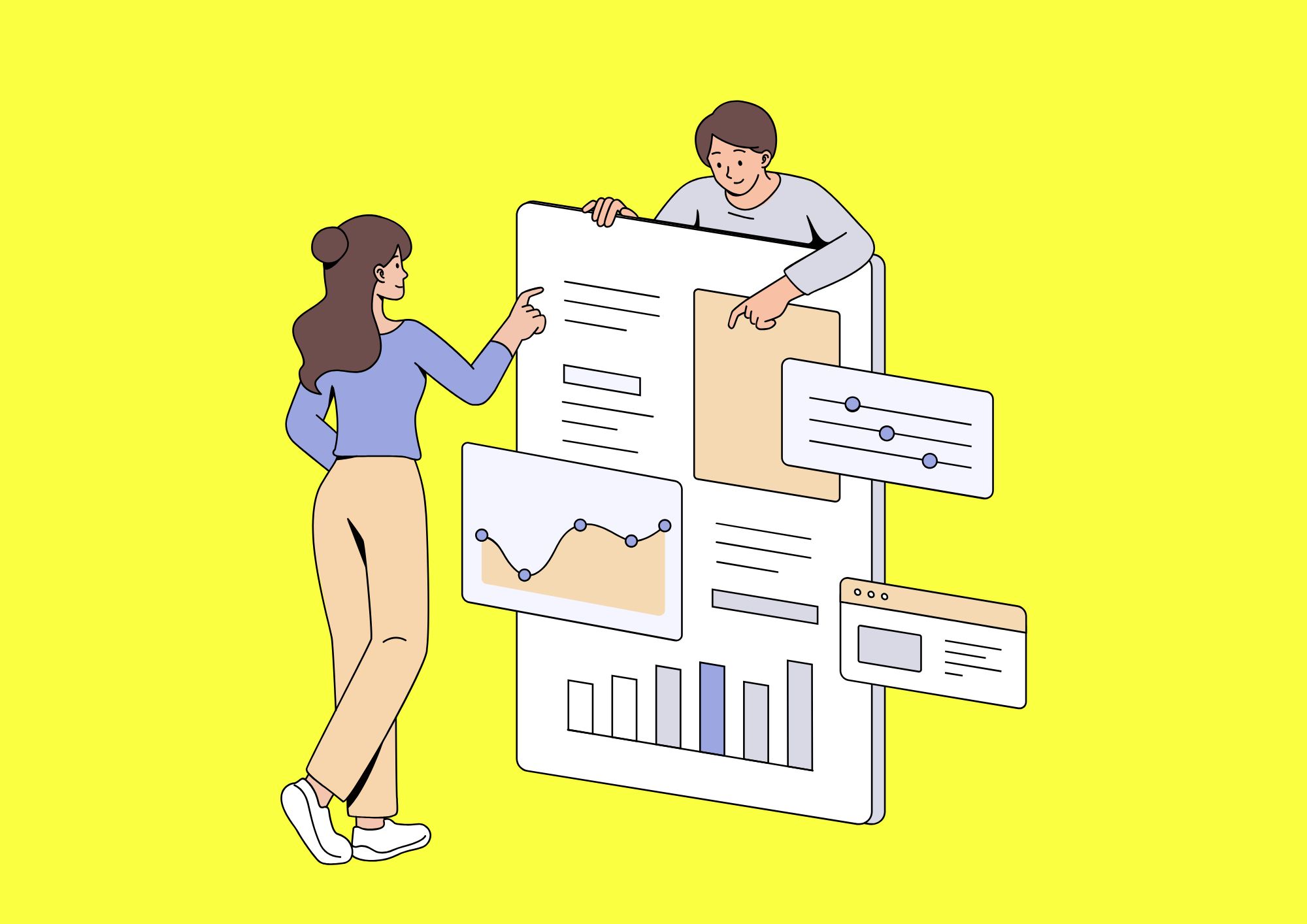
Insights and Analytics
Transform complex data into actionable insights with advanced analytics, fostering evidence-based strategies for sustainable organizational success.
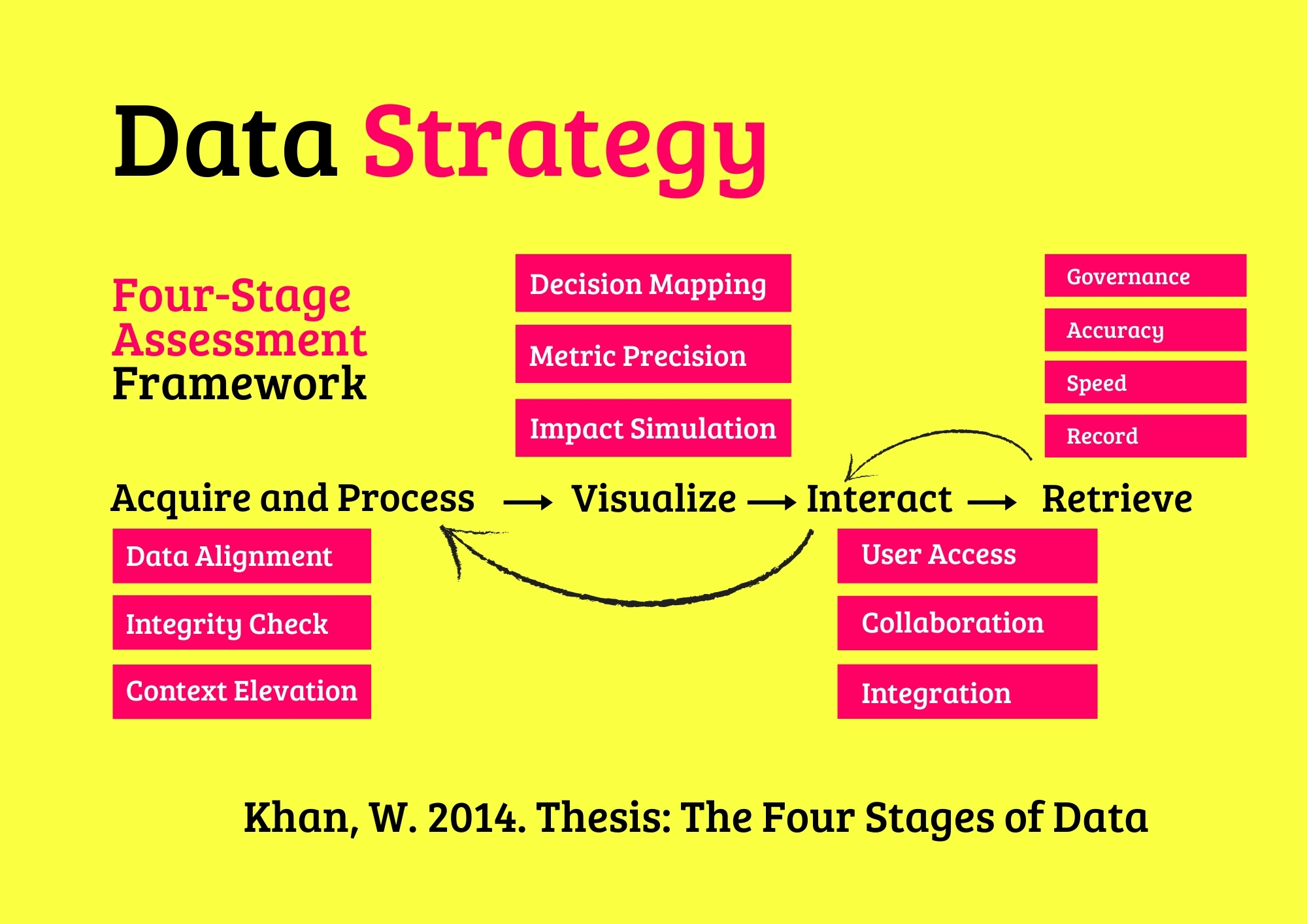
Data Strategy
Elevate your organization’s potential with our AI-enhanced data advisory services, delivering tailored strategies for sustainable success.
Explore Iconic Data Science Facts
Frequently Asked Questions
How do you help us acquire data effectively?
We assess your existing data sources and streamline collection using tools like Excel, Python, and SQL. Our process ensures clean, structured, and reliable data through automated pipelines, API integrations, and validation techniques tailored to your needs.
What’s involved in visualizing our data?
We design intuitive dashboards in Tableau, Power BI, or Looker, transforming raw data into actionable insights. Our approach includes KPI alignment, interactive elements, and advanced visual techniques to highlight trends, outliers, and opportunities at a glance.
How can we interact with our data?
We build dynamic reports in Power BI or Tableau, enabling real-time exploration. Filter, drill down, or simulate scenarios—allowing stakeholders to engage with data directly and uncover answers independently.
How do you ensure we can retrieve data quickly?
We optimize storage and queries using Looker’s semantic models, Qlik’s indexing, or cloud solutions like Snowflake. Techniques such as caching and partitioning ensure milliseconds-fast access to critical insights.
How do you assess our data strategy?
We evaluate your goals, data maturity, and gaps using frameworks like Qlik or custom scorecards. From acquisition to governance, we map a roadmap that aligns with your business impact and ROI.
What does Data Engineering entail for acquisition?
We design scalable ETL/ELT pipelines to automate data ingestion from databases, APIs, and cloud platforms. This ensures seamless integration into your systems (e.g., Excel, data lakes) while maintaining accuracy and reducing manual effort.
How do Insights and Analytics use visualization?
Beyond charts, we layer statistical models and trends into Tableau or Power BI dashboards. This turns complex datasets into clear narratives, helping teams spot patterns, correlations, and actionable strategies.
Can Data Visualisation improve interaction?
Yes. Our interactive Power BI/Tableau reports let users filter, segment, and explore data in real time. This fosters data-driven decisions by putting exploration tools directly in stakeholders’ hands.
How do you secure data during retrieval?
We implement encryption (in transit/at rest), role-based access controls (RBAC), and audit logs via Looker or Microsoft Purview. Regular penetration testing ensures compliance with GDPR, CCPA, or industry standards.
How does Machine Learning enhance data interaction?
We integrate ML models into platforms like Qlik or Power BI, enabling users to interact with predictions (e.g., customer churn, sales forecasts) and simulate "what-if" scenarios for proactive planning.
What do AI and Data Workshops teach about acquisition?
Our workshops train teams in practical data acquisition using Excel, Python, and Tableau. Topics include validation, transformation, and automation—equipping your staff with skills to handle real-world data challenges.
How do you assess which tools fit our data stages?
We analyze your workflow across acquisition, storage, analysis, and visualization. Based on your needs, we recommend tools like Power BI (visuals), Looker (modeling), or Qlik (indexing) to optimize each stage.
Can you evaluate our data retrieval speed?
Yes. We audit query performance, database design, and network latency. Solutions may include Qlik’s in-memory processing, indexing, or migrating to columnar databases for near-instant insights.
How do ongoing assessments improve visualization?
We periodically review dashboards to refine UI/UX, optimize load times, and incorporate new data sources. This ensures visuals remain relevant, performant, and aligned with evolving business goals.